
Chapter 8 Basic Regression
The fundamental premise of data modeling is to make explicit the relationship between a response variable \(y\) (also called a dependent variable or outcome variable) and an explanatory variable \(x\) (also called an independent variable, predictor variable, or covariate). Another way to state this is using mathematical terminology: we will model the outcome variable \(y\) “as a function” of the explanatory/predictor variable \(x\). When we say “function” here, we are referring to a mathematical function (not functions in R like ggplot()
).
While there exist many techniques for modeling, such as tree-based models and neural networks, in this book we’ll focus on one particular technique: linear regression. Linear regression is one of the most commonly-used and easy-to-understand approaches to modeling.
Linear regression involves a numerical outcome variable \(y\) and explanatory variables \(x\) that are either numerical or categorical. Furthermore, the relationship between \(y\) and \(x\) is assumed to be linear, or in other words, a line. However, we’ll see that what constitutes a “line” will vary depending on the nature of your explanatory variables \(x\).
In Chapter 8 on basic regression, we’ll only consider models with a single explanatory variable \(x\). In Section 8.1, the explanatory variable will be numerical. This scenario is known as simple linear regression. In Section 8.2, the explanatory variable will be categorical.
In Chapter 9 on inference for regression, we’ll revisit our regression models and analyze the results using the tools for statistical inference developed in Chapters 5, 6, and 7 on sampling, bootstrapping and confidence intervals, and hypothesis testing and \(p\)-values, respectively.
In Chapter 10 on multiple regression, we’ll extend the ideas behind basic regression and consider models with two explanatory variables \(x_1\) and \(x_2\). In Section 10.1, we’ll have one numerical and one categorical variable. In Section 10.2, we’ll have two categorical explanatory variables. In particular, we’ll consider two such models: interaction and parallel slopes models.
Modeling for explanation or prediction
Why do we have two different labels, explanatory and predictor, for the variable \(x\)? That’s because even though the two terms are often used interchangeably, roughly speaking data modeling serves one of two purposes:
- Modeling for explanation: When you want to explicitly describe and quantify the relationship between the outcome variable \(y\) and a set of explanatory variables \(x\), determine the significance of any relationships, have measures summarizing these relationships, and possibly identify any causal relationships between the variables.
- Modeling for prediction: When you want to predict an outcome variable \(y\) based on the information contained in a set of predictor variables \(x\). Unlike modeling for explanation, however, you don’t care so much about understanding how all the variables relate and interact with one another, but rather only whether you can make good predictions about \(y\) using the information in \(x\).
For example, say you are interested in an outcome variable \(y\) of whether patients develop lung cancer and information \(x\) on their risk factors, such as smoking habits, age, and socioeconomic status. If we are modeling for explanation, we would be interested in both describing and quantifying the effects of the different risk factors. One reason could be that you want to design an intervention to reduce lung cancer incidence in a population, such as targeting smokers of a specific age group with advertising for smoking cessation programs. If we are modeling for prediction, however, we wouldn’t care so much about understanding how all the individual risk factors contribute to lung cancer, but rather only whether we can make good predictions of which people will contract lung cancer.
We’ll generally focus on modeling for explanation and hence refer to \(x\) as explanatory variables. If you are interested in learning about modeling for prediction, we suggest you check out books and courses on the field of machine learning such as An Introduction to Statistical Learning with Applications in R (ISLR) (James et al. 2017).
Chapter Learning Objectives
At the end of this chapter, you should be able to…
• Calculate the correlation coefficient to estimate the strength of
association between two variables.
• Interpret a regression table to estimate the intercept and slope of a
simple regression line.
• Compute the fitted values and residuals for observed values.
• Interpret a regression table to estimate the baseline and offset
values of linear regression with a categorical explanatory
variable.
• Explain how correlation can occur without causation.
Needed packages
Let’s now load all the packages needed for this chapter (this assumes you’ve already installed them). In this chapter, we introduce the moderndive
package of datasets and functions for tidyverse-friendly introductory linear regression.
If needed, read Section 1.3 for information on how to install and load R packages.
Let’s now begin with basic regression, which refers to linear regression models with a single explanatory variable \(x\). We’ll also discuss important statistical concepts like the correlation coefficient, that “correlation isn’t necessarily causation,” and what it means for a line to be “best-fitting.”
8.1 One numerical explanatory variable
Determining the age of lions living in the wild can be difficult to estimate. One hypothesis is that the amount of black pigment in the nose increases with age and that therefore the proportion of black in the nose can be used to estimate a lion’s age. Here we’ll look at the LionNoses
data set in the abd
package. More information, including a reference to the original study, can be found by typing ?LionNoses
in the console to view its help file.
Researchers at the University of Minnesota tried to answer the following research question: can the age of male lions be used to explain the proportion of black in the nose or is there no relation? To this end, they used age and nose coloration data from 32 lions. We’ll answer these questions by modeling the relationship between proportion black and age using simple linear regression where we have:
- A numerical outcome variable \(y\) (the relative nose coloration) and
- A single numerical explanatory variable \(x\) (the lion’s age).
8.1.1 Exploratory data analysis
The data on the 32 male lions can be found in the LionNoses
data frame included in the abd
package. Recall that a crucial step before doing any kind of analysis or modeling is performing an exploratory data analysis, or EDA for short. EDA gives you a sense of the distributions of the individual variables in your data, whether any potential relationships exist between variables, whether there are outliers and/or missing values, and (most importantly) how to build your model. Let’s review the three common steps in EDA:
- Most crucially, look at the raw data values.
- Compute summary statistics (e.g., mean).
- Create data visualizations.
Let’s first look at the raw data values in the LionNoses
data set using RStudio’s spreadsheet viewer or by using the glimpse()
function as introduced in Subsection 1.4.3 on exploring data frames. Remember that getting an early sense of what your raw data looks like can often prevent larger issues down the road.
Rows: 32
Columns: 2
$ age <dbl> 1.1, 1.5, 1.9, 2.2, 2.6, 3.2, 3.2, 2.9, 2.4, 2.1, 1.9…
$ proportion.black <dbl> 0.21, 0.14, 0.11, 0.13, 0.12, 0.13, 0.12, 0.18, 0.23,…
We can see that there are two variables in LionNoses
:
proportion.black
: A numerical variable of the relative coloration of the nose. This is the outcome variable \(y\) of interest.age
: A numerical variable of the male lion’s age. This will be the explanatory variable \(x\).
An alternative way to look at the raw data values is by choosing a random sample of the rows in LionNoses
by piping it into the sample_n()
function from the dplyr
package. Here we set the size
argument to be 5
, indicating that we want a random sample of 5 rows. We display the results in Table 8.1. Note that due to the random nature of the sampling, you will likely end up with a different subset of 5 rows.
age | proportion.black |
---|---|
2.6 | 0.12 |
1.1 | 0.21 |
1.9 | 0.15 |
7.1 | 0.37 |
3.8 | 0.42 |
Now that we’ve looked at the raw values in our LionNoses
data frame and got a preliminary sense of the data, let’s move on to the next common step in an exploratory data analysis: computing summary statistics for our numerical outcome variable denoted as proportion.black
and our numerical explanatory variable age
. We can do this by using the dplyr::summarize()
function as we saw in Section 3.5, but instead let’s use the convenient skim()
function from the skimr
package that you saw earlier in Section 3.5. This function takes in a data frame, “skims” it, and returns commonly used summary statistics. Let’s take the LionNoses
data frame, with the outcome and explanatory variables proportion.black
and age
, and pipe them into the skim()
function:
── Data Summary ────────────────────────
Values
Name Piped data
Number of rows 32
Number of columns 2
_______________________
Column type frequency:
numeric 2
________________________
Group variables None
── Variable type: numeric ─────────────────────────────────────────────────────────────────────────────────────────
skim_variable n_missing complete_rate mean sd p0 p25 p50 p75
1 age 0 1 4.31 2.68 1.1 2.18 3.5 5.85
2 proportion.black 0 1 0.322 0.199 0.1 0.165 0.265 0.432
p100
13.1
0.79
Looking at this output, we can see how the values of both variables distribute. For example, the mean nose coloration was 0.322 black, whereas the mean age was 4.31 years. Furthermore, the middle 50% of nose coloration was between 0.165 and 0.433 (the first and third quartiles, or IQR), whereas the middle 50% of age falls within 2.18 to 5.85 years.
8.1.1.1 Correlation coefficient
The skim()
function only returns what are known as univariate summary statistics: functions that take a single variable and return some numerical summary of that variable. However, there also exist bivariate summary statistics: functions that take in two variables and return some summary of those two variables. In particular, when the two variables are numerical, we can compute the correlation coefficient. Generally speaking, coefficients are quantitative expressions of a specific phenomenon. A correlation coefficient is a quantitative expression of the strength of the linear relationship between two numerical variables. Its value ranges between -1 and 1 where:
- -1 indicates a perfect negative relationship: As one variable increases, the value of the other variable tends to go down, following a straight line.
- 0 indicates no relationship: The values of both variables go up/down independently of each other.
- +1 indicates a perfect positive relationship: As the value of one variable goes up, the value of the other variable tends to go up as well in a linear fashion.
Figure 8.1 gives examples of 9 different correlation coefficient values for hypothetical numerical variables \(x\) and \(y\). For example, observe in the top right plot that for a correlation coefficient of -0.75 there is a negative linear relationship between \(x\) and \(y\), but it is not as strong as the negative linear relationship between \(x\) and \(y\) when the correlation coefficient is -0.9 or -1.
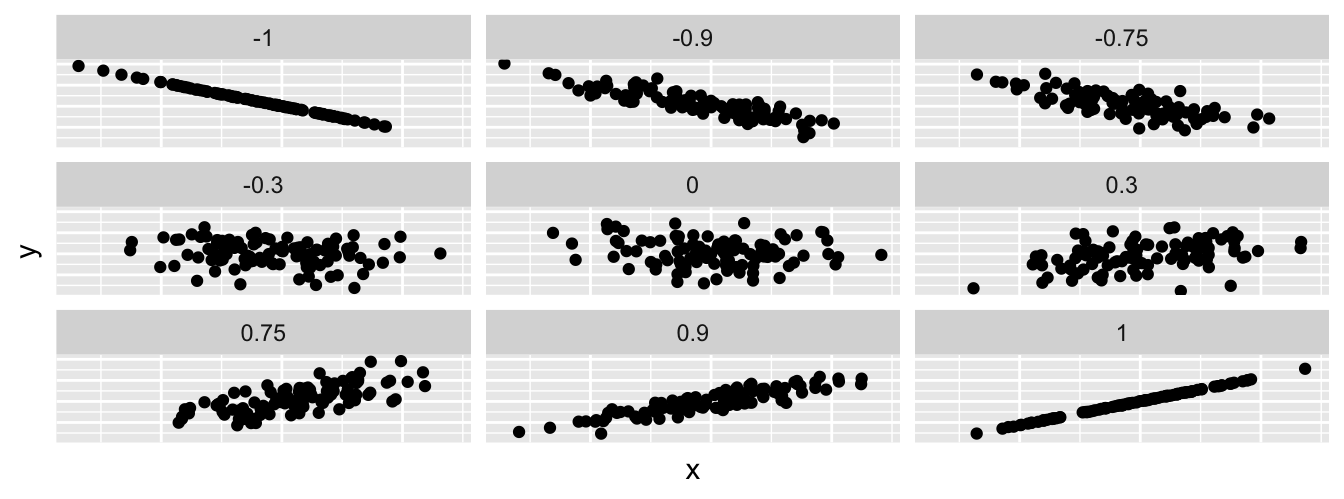
FIGURE 8.1: Nine different correlation coefficients.
The correlation coefficient can be computed using the get_correlation()
function in the moderndive
package. In this case, the inputs to the function are the two numerical variables for which we want to calculate the correlation coefficient.
We put the name of the outcome variable on the left-hand side of the ~
“tilde” sign, while putting the name of the explanatory variable on the right-hand side. This is known as R’s formula notation, which we first saw in Subsection 6.4.2. We will use this same “formula” syntax with regression later in this chapter.
cor
1 0.79
An alternative way to compute correlation is to use the cor()
summary function within a summarize()
command:
In our case, the correlation coefficient of 0.79 indicates that the relationship between proportion black and age is “clearly positive.” There is a certain amount of subjectivity in interpreting correlation coefficients, especially those that aren’t close to the extreme values of -1, 0, and 1. To develop your intuition about correlation coefficients, play the “Guess the Correlation” 1980’s style video game called “Guess the Correlation”, at http://guessthecorrelation.com/.
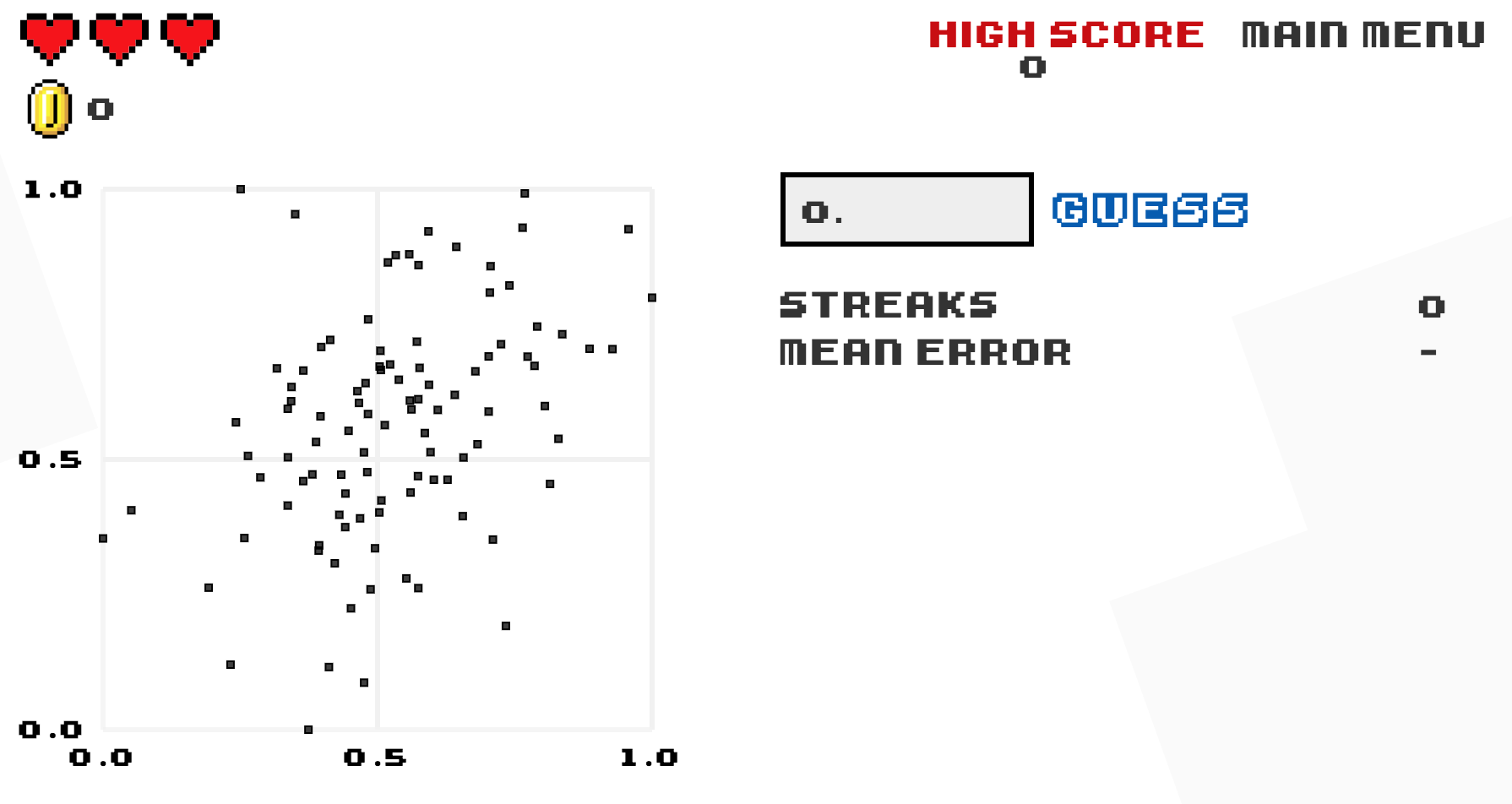
FIGURE 8.2: Preview of “Guess the Correlation” game.
Let’s now perform the last of the steps in an exploratory data analysis: creating data visualizations. Since both the proportion.black
and age
variables are numerical, a scatterplot is an appropriate graph to visualize this data. Let’s do this using geom_point()
and display the result in Figure 8.3.
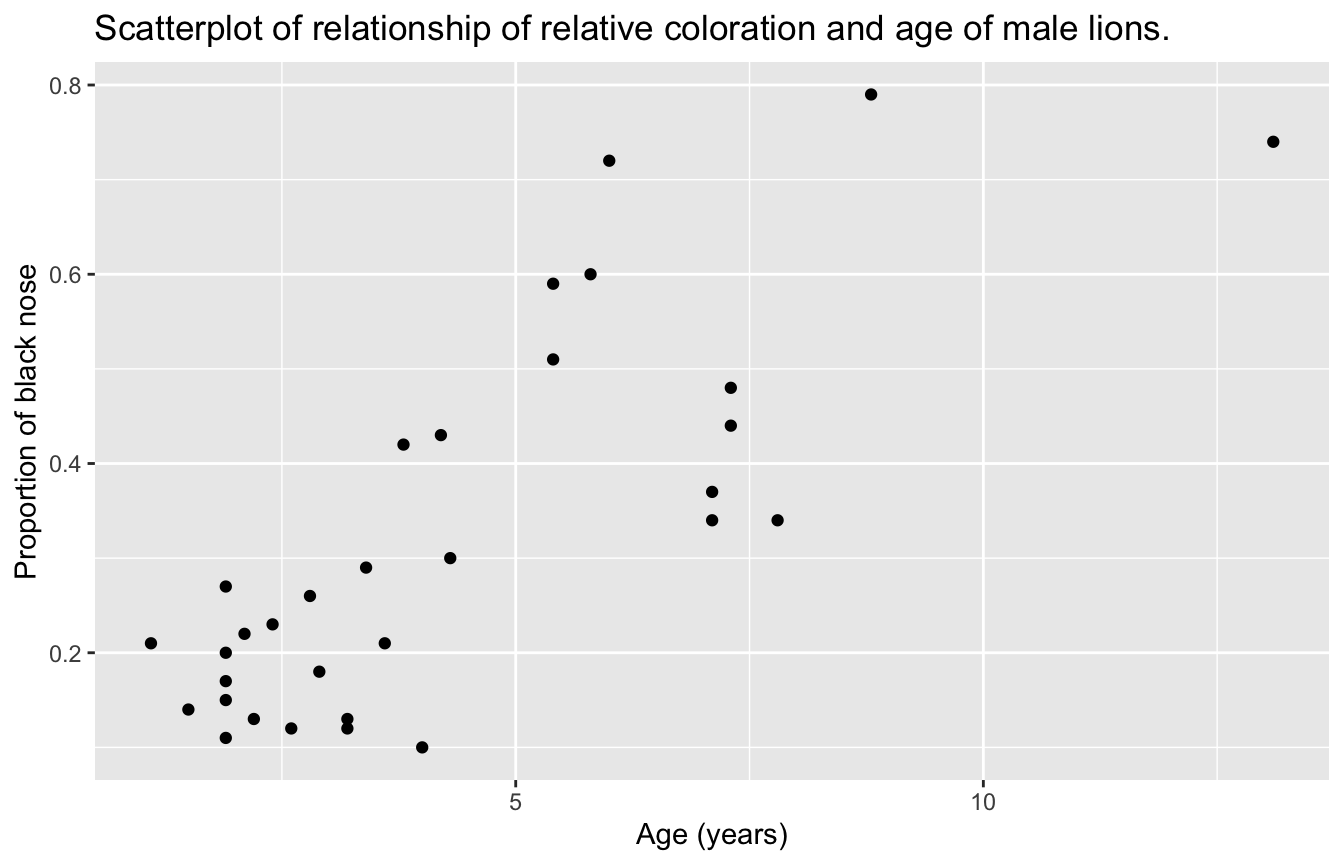
FIGURE 8.3: Lion age and nose coloration.
Observe that most ages lie between 1 and 5 years, while most nose coloration proportions lie between 0.1 and 0.3 black. Furthermore, while opinions may vary, it is our opinion that the relationship between proportion black and age is “clearly positive.” This is consistent with our earlier computed correlation coefficient of 0.79.
Let’s build on the scatterplot in Figure 8.3 by adding a “best-fitting” line: of all possible lines we can draw on this scatterplot, it is the line that “best” fits through the cloud of points. We do this by adding a new geom_smooth(method = "lm", se = FALSE)
layer to the ggplot()
code that created the scatterplot in Figure 8.3. The method = "lm"
argument sets the line to be a “l
inear m
odel.” The se = FALSE
argument suppresses standard error uncertainty bars. (We defined the concept of standard error earlier in Subsection 5.3.2.)
ggplot(LionNoses, aes(x = age, y = proportion.black)) +
geom_point() +
labs(x = "Age (years)",
y = "Proportion of black nose",
title = "Scatterplot of relationship of relative coloration and age of male lions.") +
geom_smooth(method = "lm", se = FALSE)
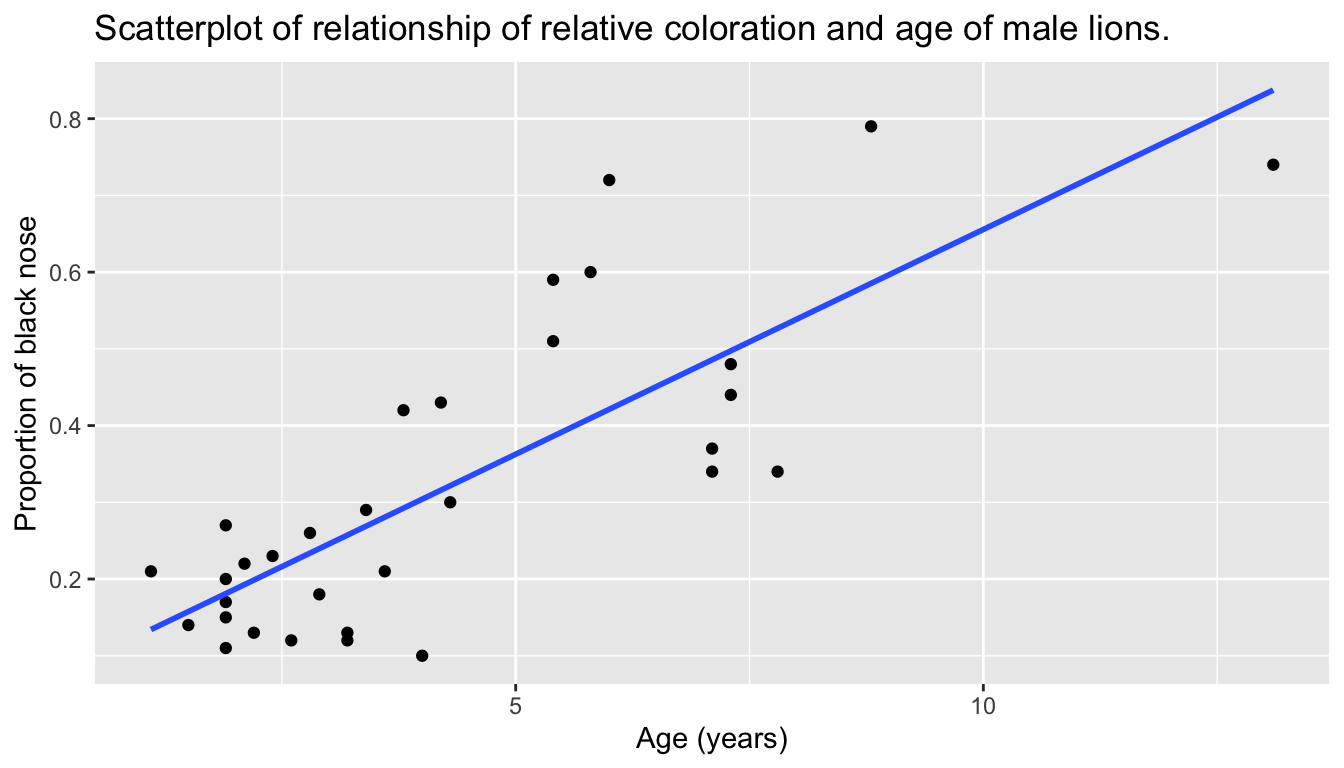
FIGURE 8.4: Regression line.
The line in the resulting Figure 8.4 is called a “regression line.” The regression line is a visual summary of the relationship between two numerical variables, in our case the outcome variable proportion.black
and the explanatory variable age
. The positive slope of the blue line is consistent with our earlier observed correlation coefficient of 0.79 suggesting that there is a positive relationship between these two variables: as lions have higher ages, they also have noses with a higher proportion of black. We’ll see later, however, that while the correlation coefficient and the slope of a regression line always have the same sign (positive or negative), they typically do not have the same value.
Furthermore, a regression line is “best-fitting” in that it minimizes some mathematical criteria. We present these mathematical criteria in Subsection 8.3.2, but we suggest you read this subsection only after first reading the rest of this section on regression with one numerical explanatory variable.
Learning check
(LC8.1) Conduct a new exploratory data analysis with the ProgesteroneExercise
data set in the abd
package. Remember, this involves three things:
- Looking at the raw data values.
- Computing summary statistics.
- Creating data visualizations.
The outcome variable \(y\) is ventilation
rate and the explanatory variable \(x\) is progesterone
levels. What can you say about the relationship between progesterone levels and ventilation rate based on this exploration?
8.1.2 Simple linear regression
You may recall from secondary/high school algebra that the equation of a line is \(y = a + b\cdot x\). (Note that the \(\cdot\) symbol is equivalent to the \(\times\) “multiply by” mathematical symbol. We’ll use the \(\cdot\) symbol in the rest of this book as it is more succinct.) It is defined by two coefficients \(a\) and \(b\). The intercept coefficient \(a\) is the value of \(y\) when \(x = 0\). The slope coefficient \(b\) for \(x\) is the increase in \(y\) for every increase of one in \(x\). This is also called the “rise over run.”
However, when defining a regression line like the regression line in Figure 8.4, we use slightly different notation: the equation of the regression line is \(\widehat{y} = b_0 + b_1 \cdot x\) . The intercept coefficient is \(b_0\), so \(b_0\) is the value of \(\widehat{y}\) when \(x = 0\). The slope coefficient for \(x\) is \(b_1\), i.e., the increase in \(\widehat{y}\) for every increase of one in \(x\). Why do we put a “hat” on top of the \(y\)? It’s a form of notation commonly used in regression to indicate that we have a “fitted value,” or the value of \(y\) on the regression line for a given \(x\) value. We’ll discuss this more in the upcoming Subsection 8.1.3.
We know that the regression line in Figure 8.4 has a positive slope \(b_1\) corresponding to our explanatory \(x\) variable age
. Why? Because as lions tend to have higher age
s, so also do they tend to have higher proportion.black
noses. However, what is the numerical value of the slope \(b_1\)? What about the intercept \(b_0\)? Let’s not compute these two values by hand, but rather let’s use a computer!
We can obtain the values of the intercept \(b_0\) and the slope for age
\(b_1\) by outputting a linear regression table. This is done in two steps:
- We first “fit” the linear regression model using the
lm()
function and save it inlion_model
. - We get the regression table by applying the
get_regression_table()
function from themoderndive
package tolion_model
.
# Fit regression model:
lion_model <- lm(proportion.black ~ age, data = LionNoses)
# Get regression table:
get_regression_table(lion_model)
term | estimate | std_error | statistic | p_value | lower_ci | upper_ci |
---|---|---|---|---|---|---|
intercept | 0.070 | 0.042 | 1.66 | 0.107 | -0.016 | 0.155 |
age | 0.059 | 0.008 | 7.05 | 0.000 | 0.042 | 0.076 |
Let’s first focus on interpreting the regression table output in Table 8.2, and then we’ll later revisit the code that produced it. In the estimate
column of Table 8.2 are the intercept \(b_0\) = 0.07 and the slope \(b_1\) = 0.059 for age
. Thus the equation of the regression line in Figure 8.4 follows:
\[ \begin{aligned} \widehat{y} &= b_0 + b_1 \cdot x\\ \widehat{\text{proportion.black}} &= b_0 + b_{\text{age}} \cdot\text{age}\\ &= 0.07 + 0.059\cdot\text{age} \end{aligned} \]
The intercept \(b_0\) = 0.07 is the average proportion of black \(\widehat{y}\) = \(\widehat{\text{proportion.black}}\) for those lions that had an age
of 0. Or in graphical terms, it’s where the line intersects the \(y\) axis when \(x\) = 0. Note, however, that while the intercept of the regression line has a mathematical interpretation, it has no practical interpretation here, since observing an age
of 0 is unlikely. Furthermore, looking at the scatterplot with the regression line in Figure 8.4, no lions had an age
near 0.
Of greater interest is the slope \(b_1\) = \(b_{\text{age}}\) for age
of 0.059, as this summarizes the relationship between proportion.black
and age
variables. Note that the sign is positive, suggesting a positive relationship between these two variables, meaning lions with higher black proportions of the nose also tend to have higher ages. Recall from earlier that the correlation coefficient is 0.79. They both have the same positive sign, but have a different value. Recall further that the correlation’s interpretation is the “strength of linear association”. The slope’s interpretation is a little different:
For every increase of 1 unit in
age
, there is an associated increase of, on average, 0.059 units ofproportion.black
.
We only state that there is an associated increase and not necessarily a causal increase. For example, perhaps it’s not that higher “age” directly cause higher proportions of black per se. Instead, the following could hold true: lions with blacker noses are more likely to survive and hence have higher ages. In other words, just because two variables are strongly associated, it doesn’t necessarily mean that one causes the other. This is summed up in the often quoted phrase, “correlation is not necessarily causation.” We discuss this idea further in Subsection 8.3.1.
Furthermore, we say that this associated increase is on average 0.059 years of age
, because you might have two lions whose age
scores differ by 1 unit, but their difference in proportion black won’t necessarily be exactly 0.059. What the slope of 0.059 is saying is that across all possible lions, the average difference in proportion black between two lions whose age
differ by one is 0.059.
Now that we’ve learned how to compute the equation for the regression line in Figure 8.4 using the values in the estimate
column of Table 8.2, and how to interpret the resulting intercept and slope, let’s revisit the code that generated this table:
# Fit regression model:
lion_model <- lm(proportion.black ~ age, data = LionNoses)
# Get regression table:
get_regression_table(lion_model)
First, we “fit” the linear regression model to the data
using the lm()
function and save this as lion_model
. When we say “fit”, we mean “find the best fitting line to this data.” lm()
stands for “linear model” and is used as follows: lm(y ~ x, data = data_frame_name)
where:
y
is the outcome variable, followed by a tilde~
. In our case,y
is set toproportion.black
.x
is the explanatory variable. In our case,x
is set toage
.- Again, the combination of
y ~ x
is called a model formula. (Note the order ofy
andx
.) In our case, the model formula isproportion.black ~ age
. We saw such model formulas earlier in this chapter when we computed the correlation coefficient using theget_correlation()
function in Subsection 8.1.1. data_frame_name
is the name of the data frame that contains the variablesy
andx
. In our case,data_frame_name
is theLionNoses
data frame.
Second, we take the saved model in lion_model
and apply the get_regression_table()
function from the moderndive
package to it to obtain the regression table in Table 8.2. This function is an example of what’s known in computer programming as a wrapper function. They take other pre-existing functions and “wrap” them into a single function that hides its inner workings. This concept is illustrated in Figure 8.5.
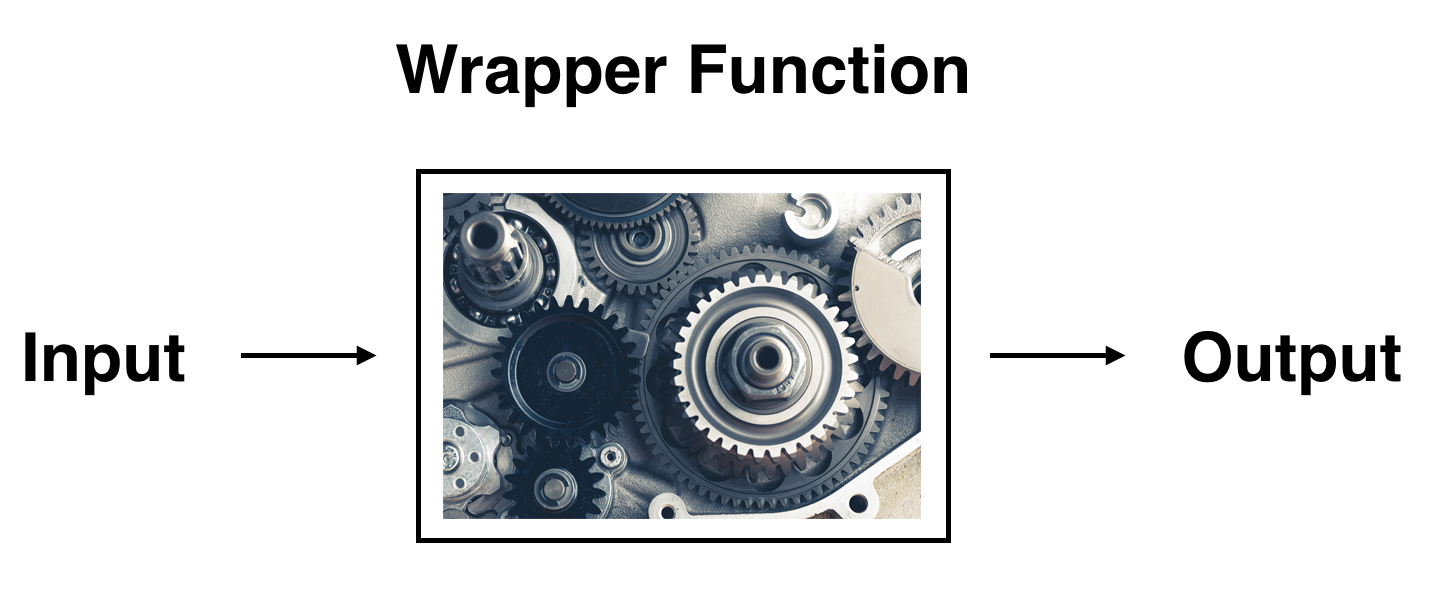
FIGURE 8.5: The concept of a wrapper function.
So all you need to worry about is what the inputs look like and what the outputs look like; you leave all the other details “under the hood of the car.” In our regression modeling example, the get_regression_table()
function takes a saved lm()
linear regression model as input and returns a data frame of the regression table as output. If you’re interested in learning more about the get_regression_table()
function’s inner workings, check out Subsection 8.3.3.
Lastly, the remaining five columns in Table 8.2 are: std_error
, statistic
, p_value
, lower_ci
and upper_ci
are the standard error, test statistic, p-value, lower 95% confidence interval bound, and upper 95% confidence interval bound. As you know, they tell us about both the statistical significance and practical significance of our results, that is, the “meaningfulness” of our results from a statistical perspective. Let’s put aside these ideas for now and revisit them in Chapter 9 on (statistical) inference for regression.
Learning check
(LC8.2) Fit a new simple linear regression using lm(ventilation ~ progesterone, data = ProgesteroneExercise)
. Get information about the “best-fitting” line from the regression table by applying the get_regression_table()
function. How do the regression results match up with the results from your earlier exploratory data analysis of this dataset?
8.1.3 Observed/fitted values and residuals
We just saw how to get the value of the intercept and the slope of a regression line from the estimate
column of a regression table generated by the get_regression_table()
function. Now instead say we want information on individual observations. For example, let’s focus on the 21st of the 32 courses in the LionNoses
data frame in Table 8.3:
age | proportion.black |
---|---|
5.8 | 0.6 |
What is the value \(\widehat{y}\) on the regression line corresponding to this lion’s age
of 5.8 years? In Figure 8.6 we mark three values corresponding to the 21st lion and give their statistical names:
- Circle: The observed value \(y\) = 0.6 is this lion’s actual proportion of the nose that was black.
- Square: The fitted value \(\widehat{y}\) is the value on the regression line for \(x\) =
age
= 5.8. This value is computed using the intercept and slope in the previous regression table:
\[\widehat{y} = b_0 + b_1 \cdot x = 0.07 + 0.059 \cdot 5.8 = 0.41\]
- Arrow: The length of this arrow is the residual and is computed by subtracting the fitted value \(\widehat{y}\) from the observed value \(y\). The residual can be thought of as a model’s error or “lack of fit” for a particular observation. In the case of this lion, it is \(y - \widehat{y}\) = 0.6 - 0.41 = 0.19.
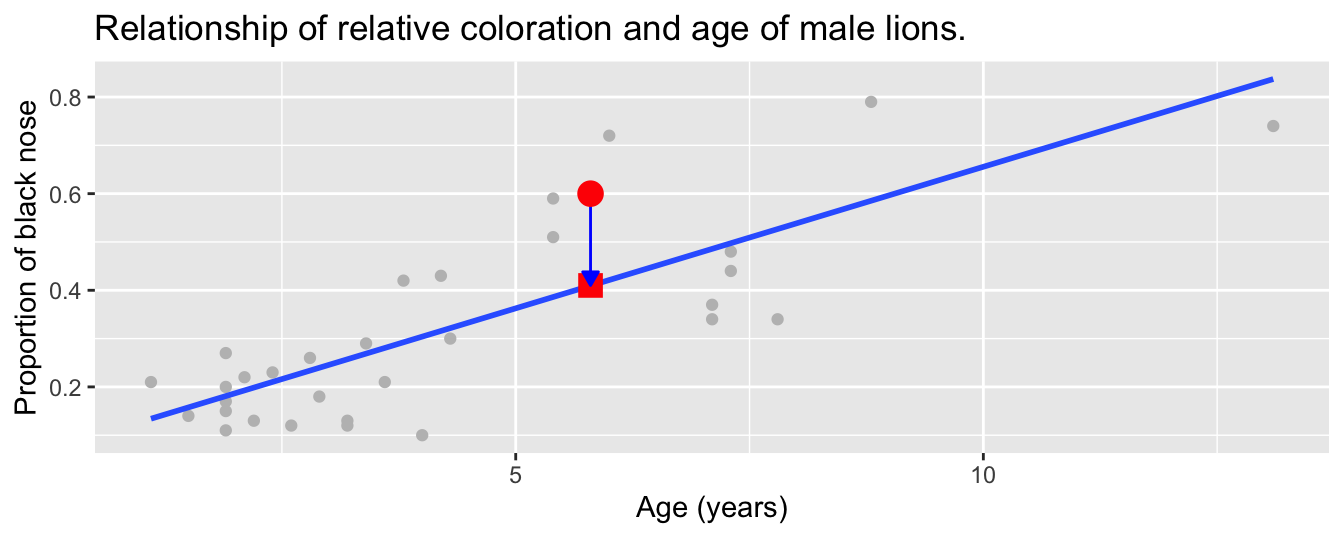
FIGURE 8.6: Example of observed value, fitted value, and residual.
Now say we want to compute both the fitted value \(\widehat{y} = b_0 + b_1 \cdot x\) and the residual \(y - \widehat{y}\) for all 32 lions in the study. Recall that each lion corresponds to one of the 32 rows in the LionNoses
data frame and also one of the 32 points in the regression plot in Figure 8.6.
We could repeat the previous calculations we performed by hand 32 times, but that would be tedious and time consuming. Instead, let’s do this using a computer with the get_regression_points()
function. Just like the get_regression_table()
function, the get_regression_points()
function is a “wrapper” function. However, this function returns a different output. Let’s apply the get_regression_points()
function to lion_model
, which is where we saved our lm()
model in the previous section. In Table 8.4 we present the results for only the 21st through 24th lions for brevity’s sake.
ID | proportion.black | age | proportion.black_hat | residual |
---|---|---|---|---|
21 | 0.60 | 5.8 | 0.410 | 0.190 |
22 | 0.72 | 6.0 | 0.421 | 0.299 |
23 | 0.29 | 3.4 | 0.269 | 0.021 |
24 | 0.10 | 4.0 | 0.304 | -0.204 |
Let’s inspect the individual columns and match them with the elements of Figure 8.6:
- The
proportion.black
column represents the observed outcome variable \(y\). This is the y-position of the 32 black points. - The
age
column represents the values of the explanatory variable \(x\). This is the x-position of the 32 black points. - The
proportion.black_hat
column represents the fitted values \(\widehat{y}\). This is the corresponding value on the regression line for the 32 \(x\) values. - The
residual
column represents the residuals \(y - \widehat{y}\). This is the 32 vertical distances between the 32 black points and the regression line.
Just as we did for the 21st lion in the LionNoses
dataset (in the first row of the table), let’s repeat the calculations for the lion of the 24th lion (in the fourth row of Table 8.4):
proportion.black
= 0.1 is the observedproportion.black
\(y\) for this lion.age
= 4.00 is the value of the explanatory variableage
\(x\) for this lion.proportion.black_hat
= 0.304 = 0.07 + 0.059 \(\cdot\) 4.00 is the fitted value \(\widehat{y}\) on the regression line for this lion.residual
= -0.204 = 0.1 - 0.304 is the value of the residual for this lion. In other words, the model’s fitted value was off by -0.204 for this lion.
At this point, you can skip ahead if you like to Subsection 8.3.2 to learn about the processes behind what makes “best-fitting” regression lines. As a primer, a “best-fitting” line refers to the line that minimizes the sum of squared residuals out of all possible lines we can draw through the points. In Section 8.2, we’ll discuss using linear regression with a numerical outcome variable and a categorical explanatory variable.
Learning check
(LC8.3) Generate a data frame of the residuals of the model lm(ventilation ~ progesterone, data = ProgesteroneExercise)
.
8.2 One categorical explanatory variable
It’s an unfortunate truth that life expectancy is not the same across all countries in the world. International development agencies are interested in studying these differences in life expectancy in the hopes of identifying where governments should allocate resources to address this problem. In this section, we’ll explore differences in life expectancy in two ways:
- Differences between continents: Are there significant differences in average life expectancy between the five populated continents of the world: Africa, the Americas, Asia, Europe, and Oceania?
- Differences within continents: How does life expectancy vary within the world’s five continents? For example, is the spread of life expectancy among the countries of Africa larger than the spread of life expectancy among the countries of Asia?
To answer such questions, we’ll use the gapminder
data frame included in the gapminder
package. This dataset has international development statistics such as life expectancy, GDP per capita, and population for 142 countries for 5-year intervals between 1952 and 2007. Recall we visualized some of this data in Figure 2.1 in Subsection 2.1.2 on the grammar of graphics.
We’ll use this data for basic regression again, but now using an explanatory variable \(x\) that is categorical, as opposed to the numerical explanatory variable model we used in the previous Section 8.1. Specifically, we’ll model the relationship between:
- A country’s life expectancy, a numerical outcome variable \(y\), and
- The continent that the country is a part of, a categorical explanatory variable \(x\).
When the explanatory variable \(x\) is categorical, the concept of a “best-fitting” regression line is a little different than the one we saw previously in Section 8.1 where the explanatory variable \(x\) was numerical. We’ll study these differences shortly in Subsection 8.2.2, but first we conduct an exploratory data analysis.
8.2.1 Exploratory data analysis
The data on the 142 countries can be found in the gapminder
data frame included in the gapminder
package. However, to keep things simple, let’s filter()
for only those observations/rows corresponding to the year 2007. Additionally, let’s select()
only the subset of the variables we’ll consider in this chapter. We’ll save this data in a new data frame called gapminder2007
:
library(gapminder)
gapminder2007 <- gapminder %>%
filter(year == 2007) %>%
select(country, lifeExp, continent, gdpPercap)
Let’s perform the first common step in an exploratory data analysis: looking at the raw data values. You can do this by using RStudio’s spreadsheet viewer or by using the glimpse()
command as introduced in Subsection 1.4.3 on exploring data frames:
Rows: 142
Columns: 4
$ country <fct> "Afghanistan", "Albania", "Algeria", "Angola", "Argentina", …
$ lifeExp <dbl> 43.8, 76.4, 72.3, 42.7, 75.3, 81.2, 79.8, 75.6, 64.1, 79.4, …
$ continent <fct> Asia, Europe, Africa, Africa, Americas, Oceania, Europe, Asi…
$ gdpPercap <dbl> 975, 5937, 6223, 4797, 12779, 34435, 36126, 29796, 1391, 336…
Observe that there are 142 rows/observations in gapminder2007
, where each row corresponds to one country. In other words, the observational unit is an individual country. Furthermore, observe that the variable continent
is of type <fct>
, which stands for factor, which is R’s way of encoding categorical variables.
A full description of all the variables included in gapminder
can be found by reading the associated help file (run ?gapminder
in the console). However, let’s fully describe only the 4 variables we selected in gapminder2007
:
country
: An identification variable of type character/text used to distinguish the 142 countries in the dataset.lifeExp
: A numerical variable of that country’s life expectancy at birth. This is the outcome variable \(y\) of interest.continent
: A categorical variable with five levels. Here “levels” correspond to the possible categories: Africa, Asia, Americas, Europe, and Oceania. This is the explanatory variable \(x\) of interest.gdpPercap
: A numerical variable of that country’s GDP per capita in US inflation-adjusted dollars that we’ll use as another outcome variable \(y\) in the Learning check at the end of this subsection.
Let’s look at a random sample of five out of the 142 countries in Table 8.5.
country | lifeExp | continent | gdpPercap |
---|---|---|---|
Togo | 58.4 | Africa | 883 |
Sao Tome and Principe | 65.5 | Africa | 1598 |
Congo, Dem. Rep. | 46.5 | Africa | 278 |
Lesotho | 42.6 | Africa | 1569 |
Bulgaria | 73.0 | Europe | 10681 |
Note that random sampling will likely produce a different subset of 5 rows for you than what’s shown. Now that we’ve looked at the raw values in our gapminder2007
data frame and got a sense of the data, let’s move on to computing summary statistics. Let’s once again apply the skim()
function from the skimr
package. Recall from our previous EDA that this function takes in a data frame, “skims” it, and returns commonly used summary statistics. Let’s take our gapminder2007
data frame, select()
only the outcome and explanatory variables lifeExp
and continent
, and pipe them into the skim()
function:
Skim summary statistics
n obs: 142
n variables: 2
── Variable type:factor
variable missing complete n n_unique top_counts
continent 0 142 142 5 Afr: 52, Asi: 33, Eur: 30, Ame: 25
ordered
FALSE
── Variable type:numeric
variable missing complete n mean sd p0 p25 p50 p75 p100
lifeExp 0 142 142 67.01 12.07 39.61 57.16 71.94 76.41 82.6
The skim()
output now reports summaries for categorical variables (Variable type:factor
) separately from the numerical variables (Variable type:numeric
). For the categorical variable continent
, it reports:
missing
,complete
, andn
, which are the number of missing, complete, and total number of values as before, respectively.n_unique
: The number of unique levels to this variable, corresponding to Africa, Asia, Americas, Europe, and Oceania.top_counts
: This refers to how many countries are in the data for each continent. In this case, the top four counts:Africa
has 52 countries,Asia
has 33,Europe
has 30, andAmericas
has 25. Not displayed isOceania
with 2 countries.ordered
: This tells us whether the categorical variable is “ordinal”: whether there is an encoded hierarchy (like low, medium, high). In this case,continent
is not ordered.
Turning our attention to the summary statistics of the numerical variable lifeExp
, we observe that the global median life expectancy in 2007 was 71.94. Thus, half of the world’s countries (71 countries) had a life expectancy less than 71.94. The mean life expectancy of 67.01 is lower, however. Why is the mean life expectancy lower than the median?
We can answer this question by performing the last of the three common steps in an exploratory data analysis: creating data visualizations. Let’s visualize the distribution of our outcome variable \(y\) = lifeExp
in Figure 8.7.
ggplot(gapminder2007, aes(x = lifeExp)) +
geom_histogram(binwidth = 5, color = "white") +
labs(x = "Life expectancy", y = "Number of countries",
title = "Histogram of distribution of worldwide life expectancies")
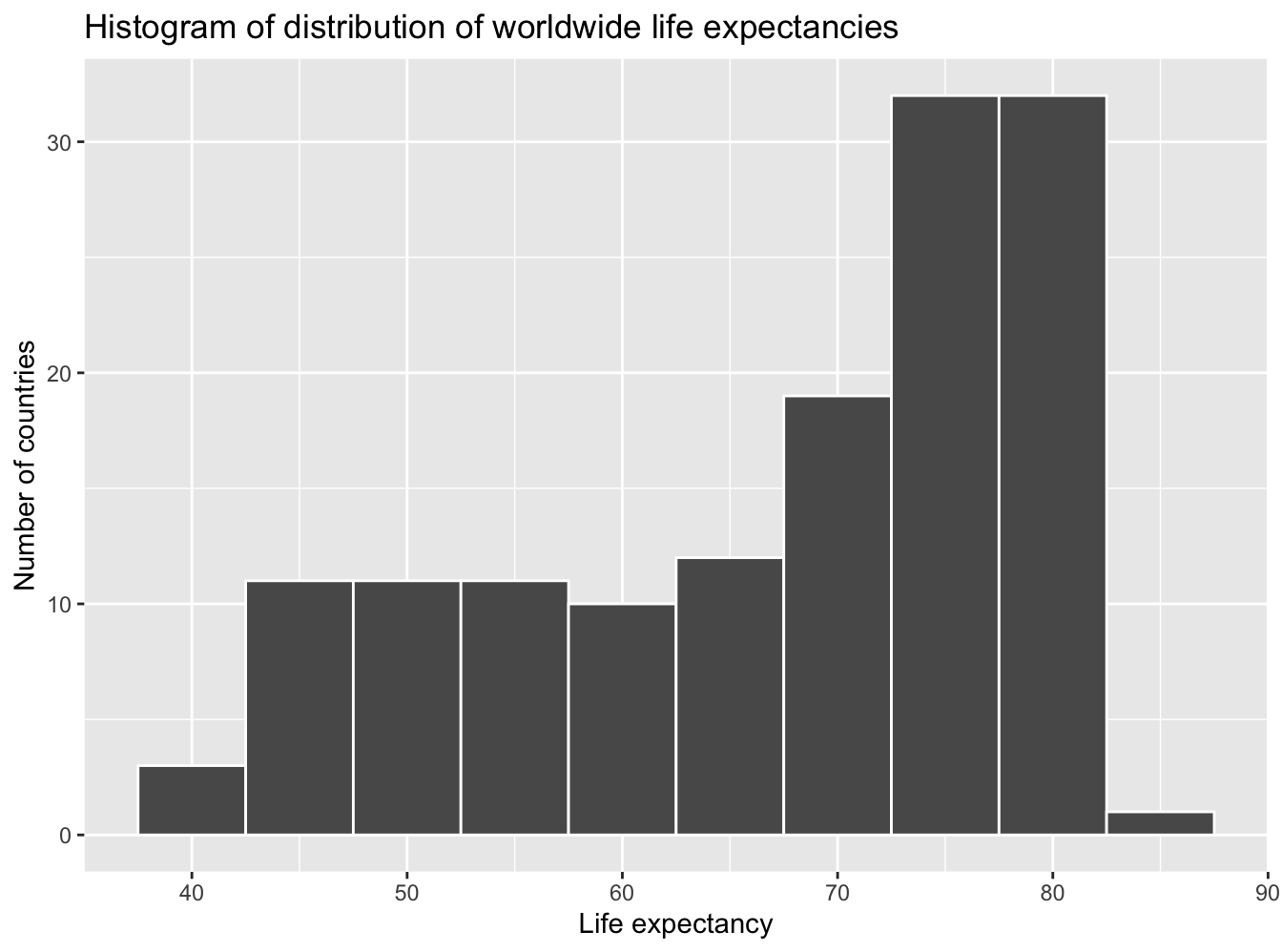
FIGURE 8.7: Histogram of life expectancy in 2007.
We see that this data is left-skewed, also known as negatively skewed: there are a few countries with low life expectancy that are bringing down the mean life expectancy. However, the median is less sensitive to the effects of such outliers; hence, the median is greater than the mean in this case.
Remember, however, that we want to compare life expectancies both between continents and within continents. In other words, our visualizations need to incorporate some notion of the variable continent
. We can do this easily with a faceted histogram. Recall from Section 2.6 that facets allow us to split a visualization by the different values of another variable. We display the resulting visualization in Figure 8.8 by adding a facet_wrap(~ continent, nrow = 2)
layer.
ggplot(gapminder2007, aes(x = lifeExp)) +
geom_histogram(binwidth = 5, color = "white") +
labs(x = "Life expectancy",
y = "Number of countries",
title = "Histogram of distribution of worldwide life expectancies") +
facet_wrap(~ continent, nrow = 2)
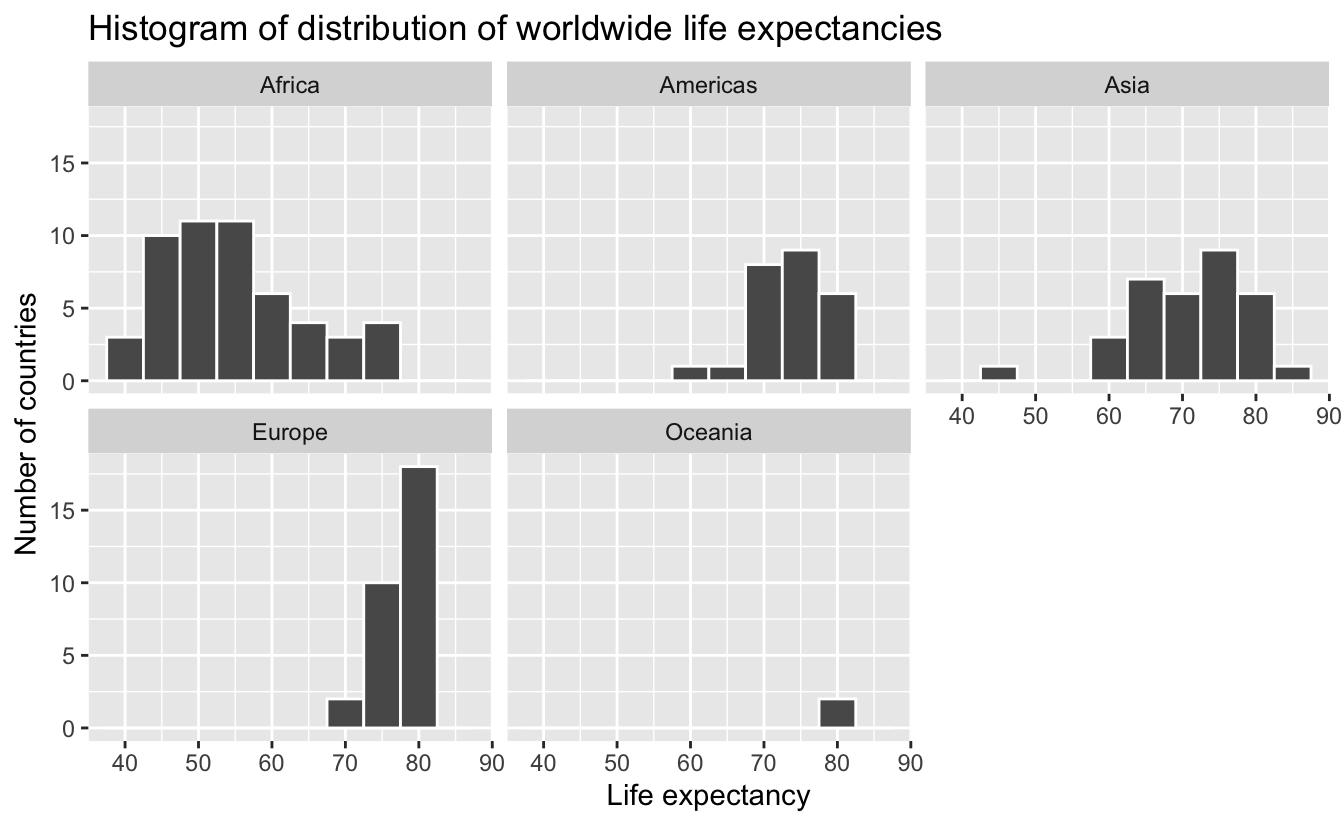
FIGURE 8.8: Life expectancy in 2007.
Observe that unfortunately the distribution of African life expectancies is much lower than the other continents, while in Europe life expectancies tend to be higher and furthermore do not vary as much. On the other hand, both Asia and Africa have the most variation in life expectancies. There is the least variation in Oceania, but keep in mind that there are only two countries in Oceania: Australia and New Zealand.
Recall that an alternative method to visualize the distribution of a numerical variable split by a categorical variable is by using a side-by-side boxplot. We map the categorical variable continent
to the \(x\)-axis and the different life expectancies within each continent on the \(y\)-axis in Figure 8.9.
ggplot(gapminder2007, aes(x = continent, y = lifeExp)) +
geom_boxplot() +
labs(x = "Continent", y = "Life expectancy",
title = "Life expectancy by continent")
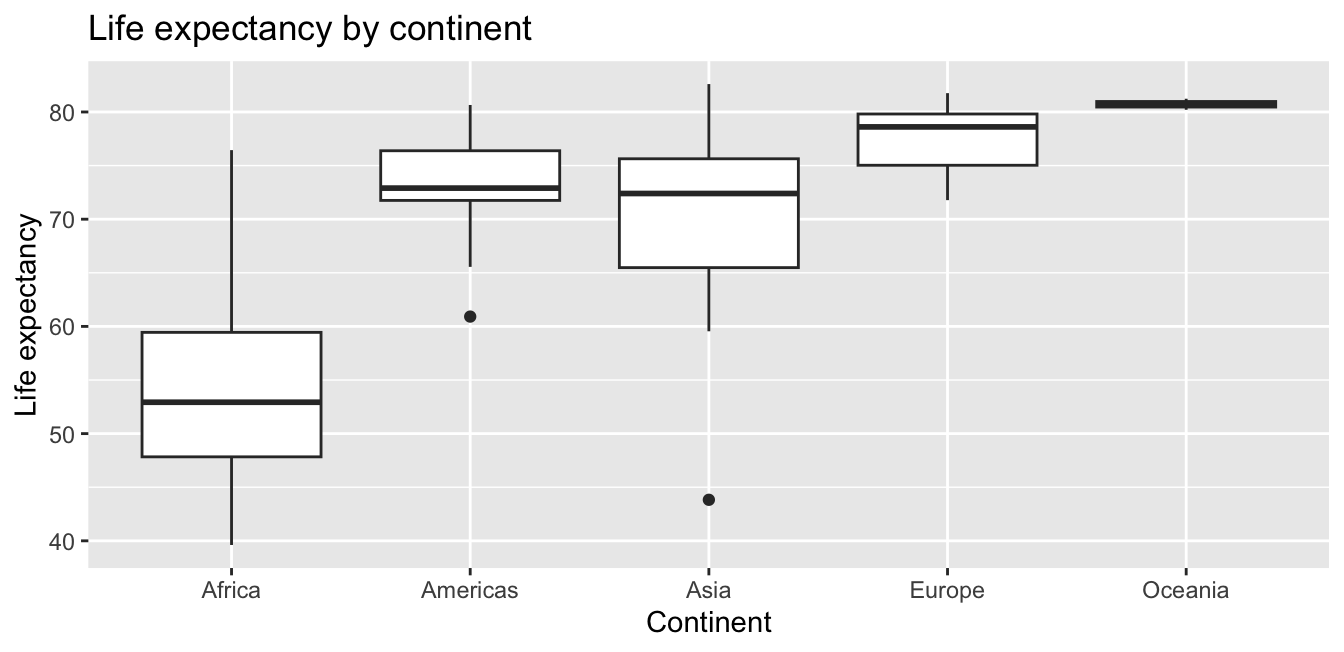
FIGURE 8.9: Life expectancy in 2007.
Some people prefer comparing the distributions of a numerical variable between different levels of a categorical variable using a boxplot instead of a faceted histogram. This is because we can make quick comparisons between the categorical variable’s levels with imaginary horizontal lines. For example, observe in Figure 8.9 that we can quickly convince ourselves that Oceania has the highest median life expectancies by drawing an imaginary horizontal line at \(y\) = 80. Furthermore, as we observed in the faceted histogram in Figure 8.8, Africa and Asia have the largest variation in life expectancy as evidenced by their large interquartile ranges (the heights of the boxes).
It’s important to remember, however, that the solid lines in the middle of the boxes correspond to the medians (the middle value) rather than the mean (the average). So, for example, if you look at Asia, the solid line denotes the median life expectancy of around 72 years. This tells us that half of all countries in Asia have a life expectancy below 72 years, whereas half have a life expectancy above 72 years.
Let’s compute the median and mean life expectancy for each continent with a little more data wrangling and display the results in Table 8.6.
lifeExp_by_continent <- gapminder2007 %>%
group_by(continent) %>%
summarize(median = median(lifeExp),
mean = mean(lifeExp))
continent | median | mean |
---|---|---|
Africa | 52.9 | 54.8 |
Americas | 72.9 | 73.6 |
Asia | 72.4 | 70.7 |
Europe | 78.6 | 77.6 |
Oceania | 80.7 | 80.7 |
Observe the order of the second column median
life expectancy: Africa is lowest, the Americas and Asia are next with similar medians, then Europe, then Oceania. This ordering corresponds to the ordering of the solid black lines inside the boxes in our side-by-side boxplot in Figure 8.9.
Let’s now turn our attention to the values in the third column mean
. Using Africa’s mean life expectancy of 54.8 as a baseline for comparison, let’s start making comparisons to the mean life expectancies of the other four continents and put these values in Table 8.7, which we’ll revisit later on in this section.
- For the Americas, it is 73.6 - 54.8 = 18.8 years higher.
- For Asia, it is 70.7 - 54.8 = 15.9 years higher.
- For Europe, it is 77.6 - 54.8 = 22.8 years higher.
- For Oceania, it is 80.7 - 54.8 = 25.9 years higher.
continent | mean | Difference versus Africa |
---|---|---|
Africa | 54.8 | 0.0 |
Americas | 73.6 | 18.8 |
Asia | 70.7 | 15.9 |
Europe | 77.6 | 22.8 |
Oceania | 80.7 | 25.9 |
Learning check
(LC8.4) Conduct a new exploratory data analysis with the same explanatory variable \(x\) being continent
but with gdpPercap
as the new outcome variable \(y\). What can you say about the differences in GDP per capita between continents based on this exploration?
8.2.2 Linear regression
In Subsection 8.1.2 we introduced simple linear regression, which involves modeling the relationship between a numerical outcome variable \(y\) and a numerical explanatory variable \(x\). In our life expectancy example, we now instead have a categorical explanatory variable continent
. Our model will not yield a “best-fitting” regression line like in Figure 8.4, but rather offsets relative to a baseline for comparison.
As we did in Subsection 8.1.2 when studying the relationship between black proportion of lion noses and age, let’s output the regression table for this model. Recall that this is done in two steps:
- We first “fit” the linear regression model using the
lm(y ~ x, data)
function and save it inlifeExp_model
. - We get the regression table by applying the
get_regression_table()
function from themoderndive
package tolifeExp_model
.
term | estimate | std_error | statistic | p_value | lower_ci | upper_ci |
---|---|---|---|---|---|---|
intercept | 54.8 | 1.02 | 53.45 | 0 | 52.8 | 56.8 |
continent: Americas | 18.8 | 1.80 | 10.45 | 0 | 15.2 | 22.4 |
continent: Asia | 15.9 | 1.65 | 9.68 | 0 | 12.7 | 19.2 |
continent: Europe | 22.8 | 1.70 | 13.47 | 0 | 19.5 | 26.2 |
continent: Oceania | 25.9 | 5.33 | 4.86 | 0 | 15.4 | 36.5 |
Let’s once again focus on the values in the term
and estimate
columns of Table 8.8. Why are there now 5 rows? Let’s break them down one-by-one:
intercept
corresponds to the mean life expectancy of countries in Africa of 54.8 years.continentAmericas
corresponds to countries in the Americas and the value +18.8 is the same difference in mean life expectancy relative to Africa we displayed in Table 8.7. In other words, the mean life expectancy of countries in the Americas is \(54.8 + 18.8 = 73.6\).continentAsia
corresponds to countries in Asia and the value +15.9 is the same difference in mean life expectancy relative to Africa we displayed in Table 8.7. In other words, the mean life expectancy of countries in Asia is \(54.8 + 15.9 = 70.7\).continentEurope
corresponds to countries in Europe and the value +22.8 is the same difference in mean life expectancy relative to Africa we displayed in Table 8.7. In other words, the mean life expectancy of countries in Europe is \(54.8 + 22.8 = 77.6\).continentOceania
corresponds to countries in Oceania and the value +25.9 is the same difference in mean life expectancy relative to Africa we displayed in Table 8.7. In other words, the mean life expectancy of countries in Oceania is \(54.8 + 25.9 = 80.7\).
To summarize, the 5 values in the estimate
column in Table 8.8 correspond to the “baseline for comparison” continent Africa (the intercept) as well as four “offsets” from this baseline for the remaining 4 continents: the Americas, Asia, Europe, and Oceania.
You might be asking at this point why was Africa chosen as the “baseline for comparison” group. This is the case for no other reason than it comes first alphabetically of the five continents; by default R arranges factors/categorical variables in alphanumeric order. You can change this baseline group to be another continent if you manipulate the variable continent
’s factor “levels” using the forcats
package. See Chapter 15 of R for Data Science (Grolemund and Wickham 2017) for examples.
Let’s now write the equation for our fitted values \(\widehat{y} = \widehat{\text{life exp}}\).
\[ \begin{aligned} \widehat{y} = \widehat{\text{life exp}} &= b_0 + b_{\text{Amer}}\cdot\mathbb{1}_{\text{Amer}}(x) + b_{\text{Asia}}\cdot\mathbb{1}_{\text{Asia}}(x) + \\ & \qquad b_{\text{Euro}}\cdot\mathbb{1}_{\text{Euro}}(x) + b_{\text{Ocean}}\cdot\mathbb{1}_{\text{Ocean}}(x)\\ &= 54.8 + 18.8\cdot\mathbb{1}_{\text{Amer}}(x) + 15.9\cdot\mathbb{1}_{\text{Asia}}(x) + \\ & \qquad 22.8\cdot\mathbb{1}_{\text{Euro}}(x) + 25.9\cdot\mathbb{1}_{\text{Ocean}}(x) \end{aligned} \]
Whoa! That looks daunting! Don’t fret, however, as once you understand what all the elements mean, things simplify greatly. First, \(\mathbb{1}_{A}(x)\) is what’s known in mathematics as an “indicator function.” It returns only one of two possible values, 0 and 1, where
\[ \mathbb{1}_{A}(x) = \left\{ \begin{array}{ll} 1 & \text{if } x \text{ is in } A \\ 0 & \text{if } \text{otherwise} \end{array} \right. \]
In a statistical modeling context, this is also known as a dummy variable. In our case, let’s consider the first such indicator variable \(\mathbb{1}_{\text{Amer}}(x)\). This indicator function returns 1 if a country is in the Americas, 0 otherwise:
\[ \mathbb{1}_{\text{Amer}}(x) = \left\{ \begin{array}{ll} 1 & \text{if } \text{country } x \text{ is in the Americas} \\ 0 & \text{otherwise}\end{array} \right. \]
Second, \(b_0\) corresponds to the intercept as before; in this case, it’s the mean life expectancy of all countries in Africa. Third, the \(b_{\text{Amer}}\), \(b_{\text{Asia}}\), \(b_{\text{Euro}}\), and \(b_{\text{Ocean}}\) represent the 4 “offsets relative to the baseline for comparison” in the regression table output in Table 8.8: continentAmericas
, continentAsia
, continentEurope
, and continentOceania
.
Let’s put this all together and compute the fitted value \(\widehat{y} = \widehat{\text{life exp}}\) for a country in Africa. Since the country is in Africa, all four indicator functions \(\mathbb{1}_{\text{Amer}}(x)\), \(\mathbb{1}_{\text{Asia}}(x)\), \(\mathbb{1}_{\text{Euro}}(x)\), and \(\mathbb{1}_{\text{Ocean}}(x)\) will equal 0, and thus:
\[ \begin{aligned} \widehat{\text{life exp}} &= b_0 + b_{\text{Amer}}\cdot\mathbb{1}_{\text{Amer}}(x) + b_{\text{Asia}}\cdot\mathbb{1}_{\text{Asia}}(x) + \\ & \qquad b_{\text{Euro}}\cdot\mathbb{1}_{\text{Euro}}(x) + b_{\text{Ocean}}\cdot\mathbb{1}_{\text{Ocean}}(x)\\ &= 54.8 + 18.8\cdot\mathbb{1}_{\text{Amer}}(x) + 15.9\cdot\mathbb{1}_{\text{Asia}}(x) + \\ & \qquad 22.8\cdot\mathbb{1}_{\text{Euro}}(x) + 25.9\cdot\mathbb{1}_{\text{Ocean}}(x)\\ &= 54.8 + 18.8\cdot 0 + 15.9\cdot 0 + 22.8\cdot 0 + 25.9\cdot 0\\ &= 54.8 \end{aligned} \]
In other words, all that’s left is the intercept \(b_0\), corresponding to the average life expectancy of African countries of 54.8 years. Next, say we are considering a country in the Americas. In this case, only the indicator function \(\mathbb{1}_{\text{Amer}}(x)\) for the Americas will equal 1, while all the others will equal 0, and thus:
\[ \begin{aligned} \widehat{\text{life exp}} &= 54.8 + 18.8\cdot\mathbb{1}_{\text{Amer}}(x) + 15.9\cdot\mathbb{1}_{\text{Asia}}(x) + 22.8\cdot\mathbb{1}_{\text{Euro}}(x) + \\ & \qquad 25.9\cdot\mathbb{1}_{\text{Ocean}}(x)\\ &= 54.8 + 18.8\cdot 1 + 15.9\cdot 0 + 22.8\cdot 0 + 25.9\cdot 0\\ &= 54.8 + 18.8 \\ & = 73.6 \end{aligned} \]
which is the mean life expectancy for countries in the Americas of 73.6 years in Table 8.7. Note the “offset from the baseline for comparison” is +18.8 years.
Let’s do one more. Say we are considering a country in Asia. In this case, only the indicator function \(\mathbb{1}_{\text{Asia}}(x)\) for Asia will equal 1, while all the others will equal 0, and thus:
\[ \begin{aligned} \widehat{\text{life exp}} &= 54.8 + 18.8\cdot\mathbb{1}_{\text{Amer}}(x) + 15.9\cdot\mathbb{1}_{\text{Asia}}(x) + 22.8\cdot\mathbb{1}_{\text{Euro}}(x) + \\ & \qquad 25.9\cdot\mathbb{1}_{\text{Ocean}}(x)\\ &= 54.8 + 18.8\cdot 0 + 15.9\cdot 1 + 22.8\cdot 0 + 25.9\cdot 0\\ &= 54.8 + 15.9 \\ & = 70.7 \end{aligned} \]
which is the mean life expectancy for Asian countries of 70.7 years in Table 8.7. The “offset from the baseline for comparison” here is +15.9 years.
Let’s generalize this idea a bit. If we fit a linear regression model using a categorical explanatory variable \(x\) that has \(k\) possible categories, the regression table will return an intercept and \(k - 1\) “offsets.” In our case, since there are \(k = 5\) continents, the regression model returns an intercept corresponding to the baseline for comparison group of Africa and \(k - 1 = 4\) offsets corresponding to the Americas, Asia, Europe, and Oceania.
Understanding a regression table output when you’re using a categorical explanatory variable is a topic those new to regression often struggle with. The only real remedy for these struggles is practice, practice, practice. However, once you equip yourselves with an understanding of how to create regression models using categorical explanatory variables, you’ll be able to incorporate many new variables into your models, given the large amount of the world’s data that is categorical. If you feel like you’re still struggling at this point, however, we suggest you closely compare Tables 8.7 and 8.8 and note how you can compute all the values from one table using the values in the other.
Learning check
(LC8.5) Fit a new linear regression using lm(gdpPercap ~ continent, data = gapminder2007)
where gdpPercap
is the new outcome variable \(y\). Get information about the “best-fitting” line from the regression table by applying the get_regression_table()
function. How do the regression results match up with the results from your previous exploratory data analysis?
8.2.3 Observed/fitted values and residuals
Recall in Subsection 8.1.3, we defined the following three concepts:
- Observed values \(y\), or the observed value of the outcome variable
- Fitted values \(\widehat{y}\), or the value on the regression line for a given \(x\) value
- Residuals \(y - \widehat{y}\), or the error between the observed value and the fitted value
We obtained these values and other values using the get_regression_points()
function from the moderndive
package. This time, however, let’s add an argument setting ID = "country"
: this is telling the function to use the variable country
in gapminder2007
as an identification variable in the output. This will help contextualize our analysis by matching values to countries.
country | lifeExp | continent | lifeExp_hat | residual |
---|---|---|---|---|
Afghanistan | 43.8 | Asia | 70.7 | -26.900 |
Albania | 76.4 | Europe | 77.6 | -1.226 |
Algeria | 72.3 | Africa | 54.8 | 17.495 |
Angola | 42.7 | Africa | 54.8 | -12.075 |
Argentina | 75.3 | Americas | 73.6 | 1.712 |
Australia | 81.2 | Oceania | 80.7 | 0.516 |
Austria | 79.8 | Europe | 77.6 | 2.180 |
Bahrain | 75.6 | Asia | 70.7 | 4.907 |
Bangladesh | 64.1 | Asia | 70.7 | -6.666 |
Belgium | 79.4 | Europe | 77.6 | 1.792 |
Observe in Table 8.9 that lifeExp_hat
contains the fitted values \(\widehat{y}\) = \(\widehat{\text{lifeExp}}\). If you look closely, there are only 5 possible values for lifeExp_hat
. These correspond to the five mean life expectancies for the 5 continents that we displayed in Table 8.7 and computed using the values in the estimate
column of the regression table in Table 8.8.
The residual
column is simply \(y - \widehat{y}\) = lifeExp - lifeExp_hat
. These values can be interpreted as the deviation of a country’s life expectancy from its continent’s average life expectancy. For example, look at the first row of Table 8.9 corresponding to Afghanistan. The residual of \(y - \widehat{y} = 43.8 - 70.7 = -26.9\) is telling us that Afghanistan’s life expectancy is a whopping 26.9 years lower than the mean life expectancy of all Asian countries. This can in part be explained by the many years of war that country has suffered.
Learning check
(LC8.6) Using either the sorting functionality of RStudio’s spreadsheet viewer or using the data wrangling tools you learned in Chapter 3, identify the five countries with the five smallest (most negative) residuals? What do these negative residuals say about their life expectancy relative to their continents’ life expectancy?
(LC8.7) Repeat this process, but identify the five countries with the five largest (most positive) residuals. What do these positive residuals say about their life expectancy relative to their continents’ life expectancy?
8.4 Conclusion
Chapter Learning Summary
-
Data modeling examines the relationship between an outcome/response
variable and one or more explanatory/predictor variables.
-
Linear regression assumes a linear relationship between a numerical
outcome variable and explanatory variables.
-
Simple linear regression assumes a linear relationship between a
numerical outcome variable and a single numerical explanatory
variable.
-
The correlation coefficient quantifies the strength of the linear
relationship between two numerical variables.
-
The regression, or best-fitting, line minimizes the distance between
the line and the observed values and can be modeled with the
mathematical equation \(\hat{y} = b_0 + b_1 \cdot x\).
-
The fitted value, \(\hat{y}\), is
the value of \(y\) on the regression
line for a given value of \(x\).
-
The residual is the difference between the fitted value \(\hat{y}\) and the observed value \(y\).
-
Basic linear regression can be extended to model the relationship
between a numerical outcome variable and a categorical
explanatory variable.
-
Linear regression with a categorical explanatory variable uses
indicator functions (or dummy variables) that return a value of 1, if
the explanatory variable is true, or 0, if not.
-
With a categorical explanatory variable, the intercept is the fitted
value of the baseline comparison group and the slope is the offset of
another group from the intercept.
-
When two variables are correlated, it doesn’t necessarily mean that
one causes the other.
-
The regression, or best-fitting, line is the line that minimizes the
sum of squared residuals.
-
A wrapper function chains together other functions to seamlessly
perform a desired function.
8.4.1 What’s to come?
In this chapter, you’ve studied the term basic regression, where you fit models that only have one explanatory variable. So far, we’ve only studied the estimate
column of all our regression tables. Chapter 9 focuses on the remaining columns: the standard error (std_error
), the test statistic
, the p_value
, and the lower and upper bounds of confidence intervals (lower_ci
and upper_ci
). Furthermore, we’ll revisit the concept of residuals \(y - \widehat{y}\) and discuss their importance when interpreting the results of a regression model. We’ll perform what is known as a residual analysis of the residual
variable of all get_regression_points()
outputs. Residual analyses allow you to verify what are known as the conditions for inference for regression.